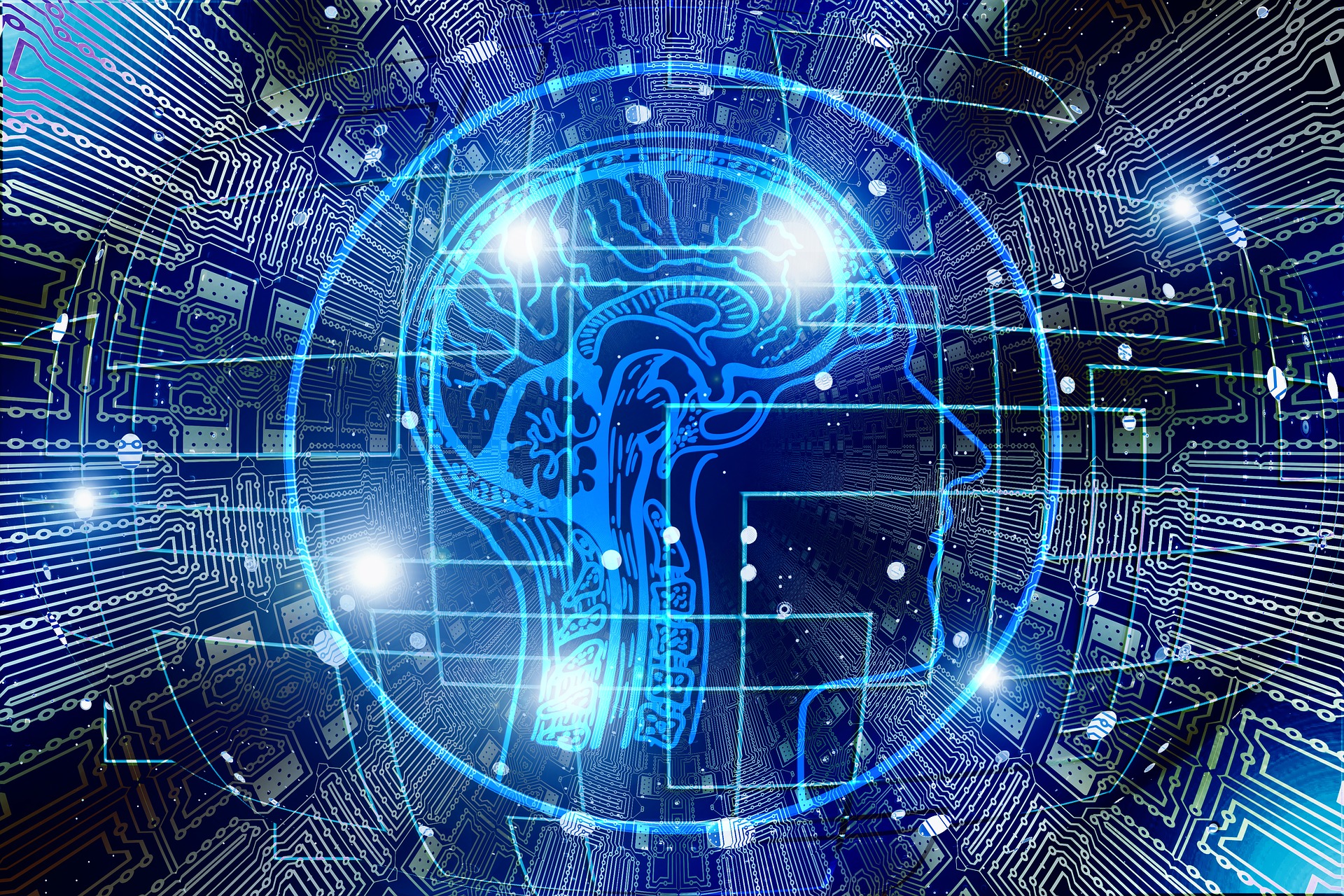
The term “AI” has at least three entirely different meanings in the modern business context, and any particular business leader usually has only one of these meanings in mind when they use the term. Not only is it common for participants in a discussion to have different interpretations of the term “AI”, but most will be unaware that this terminological confusion exists, leaving leaders in both public and private sector organisations poorly equipped to discuss one of the most important technologies of our time.
Three Types of AI
I contend that there are three distinguishable things that people mean when they use the term “AI”:
- AI as Advanced Analytics,
- AI as Applied Machine Learning, and
- AI as Future Magic.
Each of these is a valid way to view AI in the modern business context, but each leads to a radically different business strategy and technological implementation.
AI as Advanced Analytics
Category One AI is about providing interpretable information to decision-makers using increasingly-advanced, data-driven methods. This may include very sophisticated machine learning algorithms, extensive software development, and novel research, but as a business operation it occupies the same role as business intelligence. An unkind but not inaccurate aphorism would be “extreme dashboarding”.
This view of AI is neither aspirational nor transformative, but it is concrete and has the convenient feature of keeping the executive at the centre of the system. Advanced Analytics not only retains the role of the decision-maker as the lynchpin of the operations of the firm, but enhances and entrenches it. This speaks very clearly and directly to the incentives that individual leaders face within competitive organisations, and is therefore extremely attractive to them.
AI as Future Magic
Leaving aside discussion of Category Two for narrative effect, Category Three AI is speaks to the deep-seated need leaders often have for their organisation to be “more”; more innovative, more profitable, more effective, more agile, more exciting. They are the captain of a vessel that they want to turn like a speedboat but handles like a container ship. Convinced of both the power of modern technology and the wealth of data that they must surely have, the Future Magic of AI allows them to imagine a world in which they can be a visionary leader of a dynamic firm without bothering with the much more difficult work of figuring out how such a dramatic transformation could possibly be made.
It is much too easy to parody such views, but with Future Magic AI there is a complicating wrinkle that goes some way to absolving people who believe in it: many of their ideas about this Future Magic are inspired by real, present-day technology that they have seen with their own eyes. Discovering the structure of proteins with AlphaFold, generating movie scripts with GPT-3, and inventing photorealistic 3-D scenes with neural radiance fields are all real possibilities that look like Future Magic.
What’s more, this Future Magic seems to be created by people who look very much like people they know. Sure, Google DeepMind might employ people at the absolute top of their game, but they know quite a few very smart people with PhDs in String Theory and Algebraic Geometry who didn’t want (or couldn’t find) a postdoc position and now work in their Data Science team. They can read the latest research and create novel algorithms too. So if AI can do all this Future Magic, and the people who make the Future Magic are pretty similar to their people, surely they can have some Future Magic too.
Unfortunately, these quite obvious ingredients of transformative, novel AI technologies are necessary but not sufficient for the real application of Future Magic AI. These advanced technologies are not only developed by organisations with large budgets and an incredible depth of technical talent, but other systems that support the creation and deployment of new, highly complex technologies; networks of systems, processes, and cultural norms that enable these technologies to have an effect on the real world operations of the organisation. Businesses struggling with low-complexity operations in low-productivity environments are very far away from having the structures necessary to make this technology effective. To put it bluntly, one cannot put a Ferrari engine in a 1994 Honda Civic and expect to win a Grand Prix.
Moreover, many firms do not have the organisational or operational complexity to make sophisticated technologies valuable. Part of AI’s particular value proposition is its ability to manage complexity. New Zealand, where I come from, has a particularly acute case of this problem in that not only do many firms lack the kind of complexity that makes Future Magic AI invaluable, but the entire economy lacks that sophistication.
Thus, the executive who looks to Future Magic AI to solve their organisational woes is not being foolish or unreasonable; this technology does exist, it just isn’t useful or practical for their firm.
AI as Applied Machine Learning
We now come to Category Two AI. AI in the sense of Applied Machine Learning is the meat in the sandwich; it’s the advanced technology that almost any organisation can leverage to immediate and often dramatic effect. This technology is accessible, affordable, and in common use. The only problem with it is that we don’t call it AI. Instead, we call it Natural Language Processing or Computer Vision. Precisely because this technology is real, useful, and within reach, it’s not considered to be AI.
In my conversations with businesses and government agencies, I am continually trying to find better language to talk about AI in the sense of Applied Machine Learning. I have found two main roadblocks to making these conversations successful. The first is the disparate nature of the terminology; indeed, from the perspective of a business conversation it is Applied Machine Learning’s defining feature. This means that even if a fruitful conversation can be started about Computer Vision, for example, there is no logical connection in the mind of the executive between the topic we’re currently discussing and the need they have to classify their archive of audio recordings. The lack of a useful overarching term inhibits the connection of business problems to technological solutions.
The second difficulty is that Applied Machine Learning is neither as easily understandable and non-threatening as Advanced Analytics nor as inspiring and carefree as Future Magic. This makes it attractive primarily to people within the organisation whose motivation is to achieve real outcomes. These pragmatic individuals are, unfortunately, often quite distant from those holding the purse strings, which tends to reduce their ability to create organisational change and real business value.
What’s in a name?
The words that we use to describe the things we do shape, enhance, and restrict how we collectively think about our work, and therefore what it is possible to achieve in a business. The disparate interpretations that people have of the term AI, compounded by the nescience of the existence of these differences, inhibits the use of real, practical technologies and limits their potential to transform organisations. Business cultures that find more precise ways to talk about AI will reap outsized rewards and, perhaps as importantly, make for less frustrating conversations.